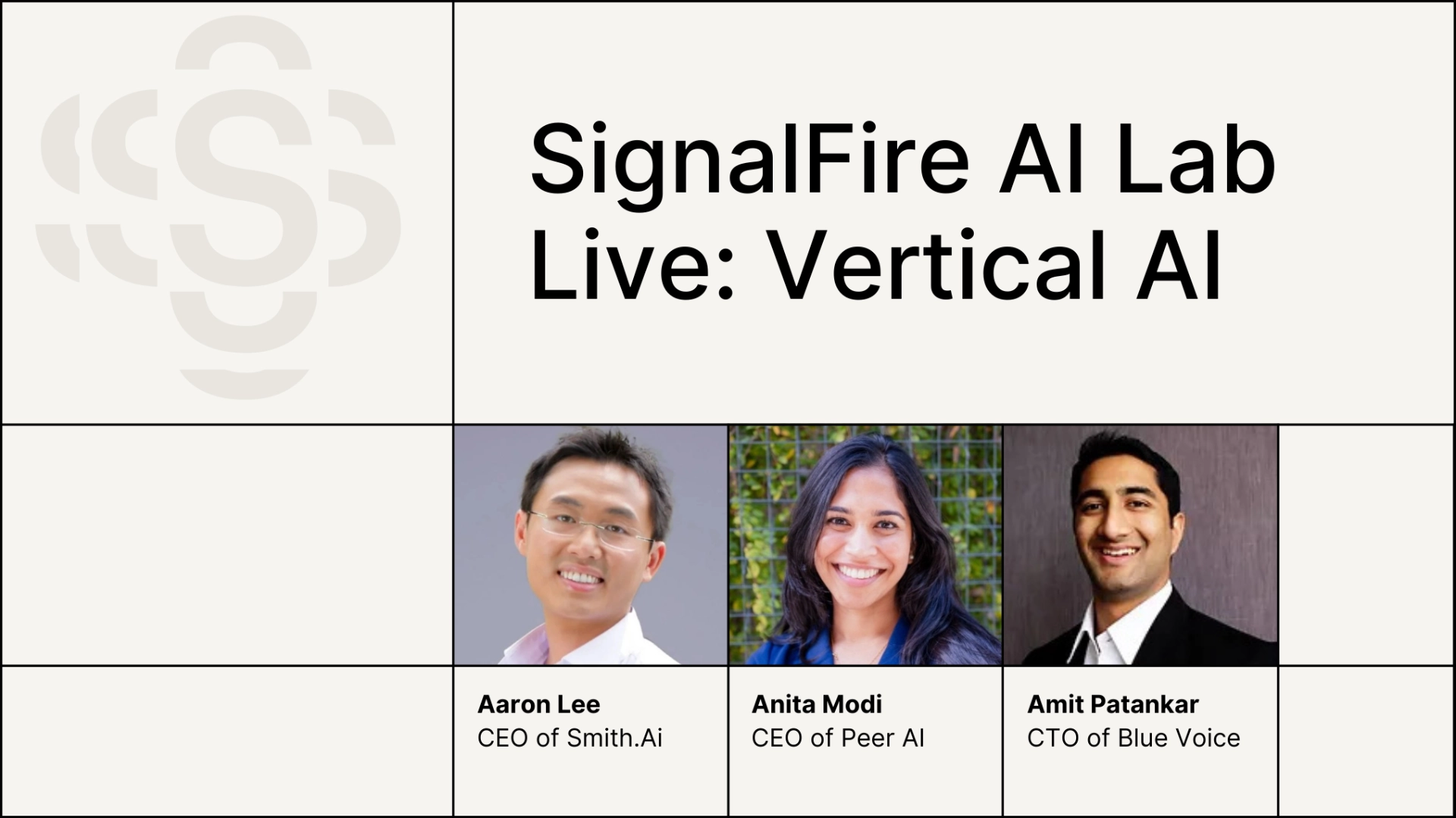
The customer is actually part of your team when you're building a vertical AI startup. You rely on them for training data and performance feedback. They shape your pricing and whether you sell software or a service. Together, you'll discover where humans must stay in the loop.
Startups that master the art of customer collaboration will build better products that grow more quickly. SignalFire brought together co-founders of three vertical AI startups to teach fellow builders what tactics are succeeding in the market:
- Aaron Lee, CEO of Smith.Ai, which powers customer support, AI receptionists, and outbound sales for SMBs
- Anita Modi, CEO of Peer AI, which uses generative AI to automate regulatory medical writing for life sciences companies
- Amit Patankar, CTO of Blue Voice, which instantly provides verified knowledge to law enforcement and first responders
Here's a summary of their top insights into how to build vertical AI startups:
- Data access: Partnering with customers can grant you access to nonpublic data that horizontal foundation models can't crawl from the open web, strengthening your data moat. You can ingest data on their customer interactions, workflows, and internal documentation, but also interview end users and experts to manually record data and rate your AI's responses. Keep in mind that the more value you can provide initially, the more trust and access they'll give you.
- Pricing models: The promise of AI is streamlining work, so selling with a seat-based pricing model may shortchange your startup. You could work with customers to find a model that works by, for instance, charging a portion of what you're saving them on headcount, vendor fees, customer acquisition, or on the added revenue you generate for them. Buyers want predictability, so it's better to sell according to a metric they intuitively understand and can estimate, such as "calls handled per day," rather than by call minute or token. Pricing flexibly based on customer size will help you avoid pricing out smaller buyers or earning too little from your biggest accounts. Focus on delivering an affordable product, and increase prices as you expand your services to align your pricing with the value you’re delivering.
- Product formats: Not all customers want to buy software-as-a-service. Your product will be much easier to adopt if it can slot into an existing solution. If a customer is used to outsourcing work to a service provider, you can sell a managed service where you return completed work, or you can sell a self-serve tool if they're more sophisticated software users. Be careful not to be dragged into building nonscalable, one-off solutions for edge cases that won't be relevant to other customers.
- Performance monitoring: There's no universal scorecard for AI, so consider building one for you and your customers to define what quality level is satisfactory. You could compare AI outputs to best-in-class human-designed templates or build companion AI systems that assess the quality of outputs compared to those from earlier versions of your product. Treat every mistake as your fault, even if it was caused by misuse of the product or imprecise training data, and see it as a chance to refine your UX or model.
- Where to keep the human in the loop: AI isn't yet ready to handle every high-stakes problem. Divide use cases into more benign tasks you can fully automate (because failure isn't catastrophic) and critical functions (for instance, when lives are at risk and you need a human to double-check). One helpful litmus test is to honestly consider whether you'd be comfortable with your AI handling your own tasks if you were a customer. If you wouldn't trust it with your family, keep a human in the loop, and look for more ways to direct performance feedback from customers back into your model.
Now, let's dig into how to put these strategies into practice with examples of what's working with customers today.
Unlocking proprietary data: The foundation of Vertical AI
Vertical AI startups face a unique challenge in acquiring the specialized data needed to train their models. Unlike large language models that can scrape the open web, vertical AI requires access to industry-specific, often confidential information.
To access sensitive clinical trial data to build its tool for automating medical writing, Peer AI leveraged its team's deep experience handling confidential life sciences information. "We invested many hours up front, went through intense security scrutiny, and put in a lot of effort to show that this data is handled appropriately and in compliance with industry standards," Modi says.
Patankar shares Blue Voice's creative solution to initial data scarcity: "In the beginning, it was a lot of manual data collection. So we would just gather public records policies from different departments, and then we'd actually hire police officers in their spare time to ask a question, and we'd give an answer, and they would rate the answer." This hands-on approach not only provided valuable training data but also ensured the relevance and accuracy of the information.
Lee highlights Smith.ai's strategy of providing immediate utility to get access to data: "We basically say, ‘Hey, we can actually help you answer your phone calls, we can answer your live chat, we can answer your SMS, we can even make outbound calls for outreach or SDR support.’ So in exchange for us accessing their conversational client data, we're providing value." This approach allows Smith.ai to generate revenue while simultaneously building its data moat.
Pricing strategies: Aligning value with customer outcomes
Traditional pricing models may not suit vertical AI products. The panelists emphasized the importance of aligning pricing with the value delivered and the customer's existing purchasing patterns. Modi explains Peer AI's approach: "There is already an amount budgeted when a trial program starts, with a line item for generating the clinical trial documents needed throughout the program. So for us, it was about plugging into this mental model and saying, ‘We’re going to charge on a per-document basis.'"
Patankar acknowledges the challenge in quantifying the value of preventive AI solutions. Blue Voice addressed this by initially offering lower prices for design partners who provided their data and increasing them with newer and more compelling features.
Lee emphasizes the importance of simplicity and predictability in pricing: "We don't price per token, right? Because they don't understand what 'token' means. We wanted to come up with something very simple and very predictable. So we charge per call as opposed to charge per minute," as that's more intuitive for their small and medium-sized business clients.
Product format: Adapting to customer workflows
The panelists stressed the importance of adapting product formats to existing customer workflows. Modi says, "For regulatory writing, biopharma companies often outsource the work. These huge organizations, CROs—contract research organizations—often price on time and materials and usually have a document price estimate." By offering a more turnkey solution for getting the work done rather than just selling a tool, Peer AI makes adoption easier for customers.
Patankar cautions against building nonscalable solutions: "The thing you want to avoid is to have these nonscalable services that your clients ask for, that might land you one client, but that are not applicable across the spectrum of your customers."
Monitoring performance: Defining and measuring quality
Without established industry standards for AI performance, vertical AI startups must create their own quality metrics. Modi shares Peer AI's approach: "We actually created an evaluation framework. We said, 'We interviewed our customers and analyzed checklists we've seen across companies, across quality, across formatting, and we've come up with the master “industry standard” framework that we think is best in class.'"

Patankar emphasizes the importance of continuous improvement: "We created our own strict model that grades us." They also focused on making their system deterministic to ensure consistency, where the same input will produce the same output without deviation.
Lee shared Smith AI's multilayered approach, using AI to evaluate AI, which can surface how an underlying foundation model update has affected accuracy. Smith AI supplements this with human evaluators, and instead of a single human, they use several to limit bias.
Human-in-the-loop: Balancing automation and expertise
While AI can handle many tasks, the panelists agreed that human oversight remains crucial, especially in high-stakes industries. Patankar explains Blue Voice uses human input to understand the difference between benign use cases around administrivia that could eventually be fully automated, and where sensitive case details and edge cases require more input from human officers.
Modi emphasizes the regulatory necessity of human involvement in the pharmaceutical industry, where human safety is on the line: " You need to ensure there's accuracy and completeness and a full review of this information before there's any sort of regulatory submission."

Many customers want the reliable accuracy of having a human in the loop, and while it's difficult to implement and scale, they are happy to pay more for it. "Some of the very big companies have the human in the loop. They just don't want to tell you about it. It doesn't sound very sexy," Lee says. "But we actually embrace the solution. Even technology with the human in the loop can drive significant cost savings.”
Keep your customers close
Powerful foundation models are ready to be fine-tuned by vertical AI startups for every industry. But it's the founders who invite customers into every part of their build-out who will see their products adopted the fastest.
If you're building a vertical AI startup, SignalFire's team would love to hear from you. We've been building AI ourselves for a decade to help source and support portfolio companies. With our AI Lab program, we connect founders with corporate partners who can provide the training data, design guidance, and initial customer base that startups need. Reach out to one of our teammates, and sign up for our vertical AI newsletter for updates and insights like these.
*Portfolio company founders listed above have not received any compensation for this feedback and may or may not have invested in a SignalFire fund. These founders may or may not serve as Affiliate Advisors, Retained Advisors, or consultants to provide their expertise on a formal or ad hoc basis. They are not employed by SignalFire and do not provide investment advisory services to clients on behalf of SignalFire. Please refer to our disclosures page for additional disclosures.
Related posts

Angel investing 101 for engineers
