
What's bigger than one trillion? The number of data points the global healthcare industry had produced by 2020. Predictions have health data generation making up 30% of all global data by 2030.
The rise of electronic health records (EHRs), new regulatory requirements, and both consumer and medical-grade wearables have triggered an explosion of raw health information.
Despite the sector generating nearly one-third of the world’s data, very little of it is being put to good use with modern AI. And while bad data quality in enterprise can mean a lost sale, in healthcare it could mean a lost life.
To power the next wave of medical AI innovations, the world needs infrastructure to clean and organize health data, make it interoperable, and safeguard its security and privacy.
In this post, we’ll lay out some of the biggest technology and regulatory shifts affecting the healthcare data space and a dozen specific opportunities where SignalFire is looking to invest in AI and Big Data in healthcare. These opportunities are split across 5 key segments:
- AI and Big Data for healthcare developers: The compliant data infra layer
- AI and Big Data for hospitals and providers: Improving the patient journey
- AI and Big Data for payors: Healthcare efficiency at scale
- AI and Big Data for pharma: Catalyzing new medicines
- AI and Big Data for patients: Affordable, transparent care
We’ll also discuss the challenges of AI in healthcare.
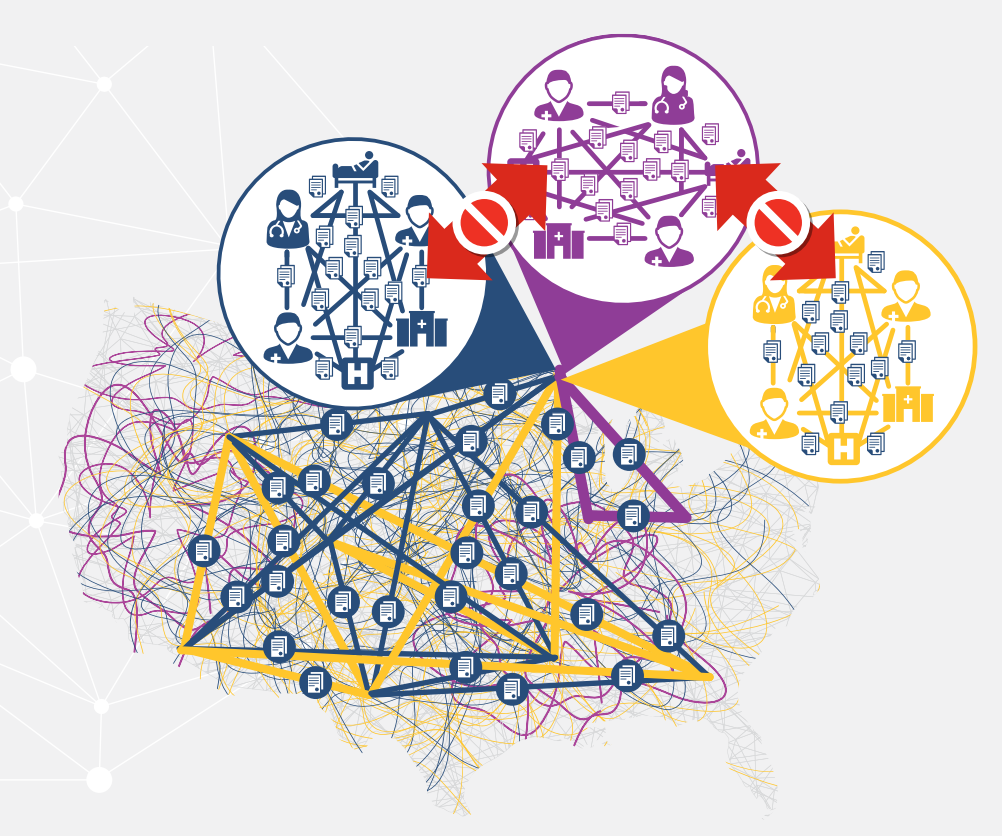
1. AI and Big Data for healthcare developers: The compliant data infra layer
With AI, it’s garbage in, garbage out, so the industry first needs infrastructure to improve data quality. Before a model or analytics can be built on top of a dataset, we need to address where to get access to raw data, how to cleanse and structure it, how to join datasets into a complete data record for each patient, and how to store it securely.
Fortunately, the Cures Act mandated the bidirectional exchange of patient clinical data through the Trusted Exchange Framework and Common Agreement (TEFCA) to address some of these problems.
Essentially, TEFCA requires every healthcare organization to make its data more accessible across states, hospitals, and provider networks so patients’ care teams always have the information they need. To be eligible to access this shared data—including 90% of U.S. patients—entities must receive the Qualified Health Information Network (QHIN) designation, which indicates they can compliantly handle data at scale.
- Developer access to interoperable data: To build new healthcare products that make use of QHIN data, developers need a compliant API layer. SignalFire portfolio company Health Gorilla is the only QHIO (Qualified Health Information Organization in California) and QHIN-approved access point for electronic medical records, lab data, and more. It’s already solved many of the raw data access, cleanliness, integration, and privacy-safe storage issues to build a technical foundation for the next generation of solutions.
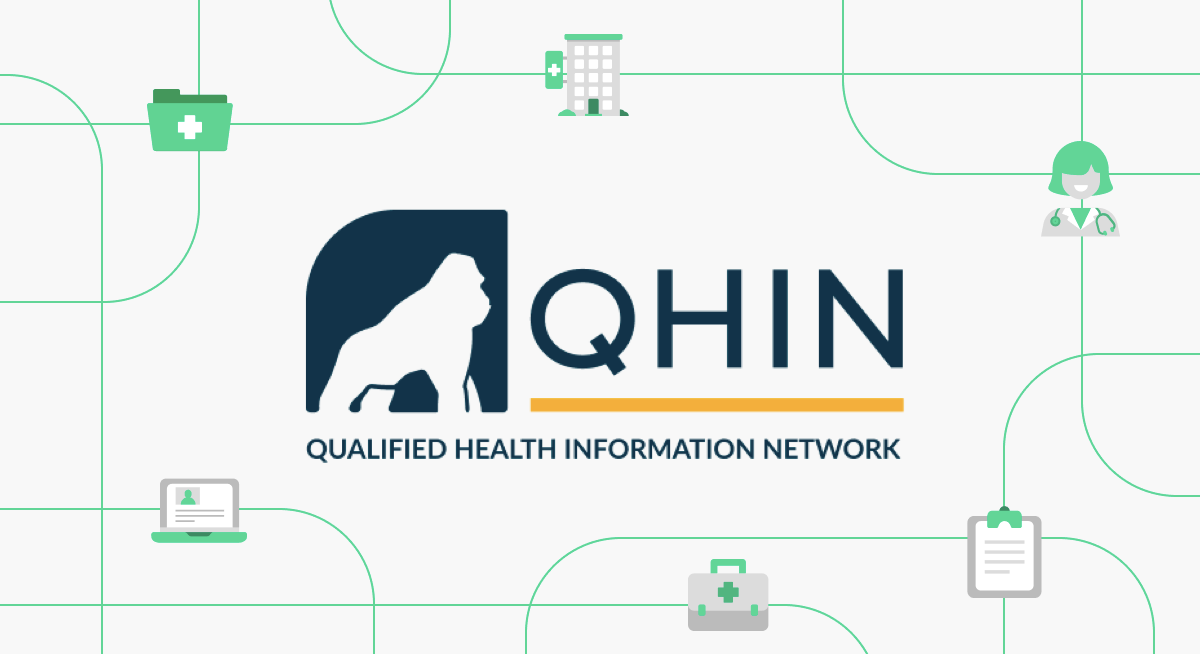
- HIPAA-compliant healthcare marketing: It’s illegal to use Facebook Ads, Google Analytics, and YouTube to market healthcare products and services if you don’t follow precise privacy regulations. SignalFire portfolio company FreshPaint offers a healthcare privacy protection platform that sits between businesses and popular marketing tools to block leaks of sensitive data, prevent noncompliant tracking, and give visitors anonymous identifiers.
2. AI and Big Data for hospitals and providers: Improving the patient journey
The broadest area of opportunity for AI in healthcare is to support and streamline those actually providing the care. They endure endless busywork while trying to make tough decisions, Meanwhile, the clinical data they produce is one of the most valuable datasets because it captures what’s needed to treat patients: symptoms, blood test results, medication history, diagnosis, care plan, side effects, and ongoing progress. Here are the areas where SignalFire is particularly excited:

- Personalized patient engagement: Knowing everything we know about a patient’s medical history, demographic information, and consumer preferences, how do we proactively engage with them in a way that encourages them to come in for preventive visits, obtain further education on conditions they may be at higher risk for, provide education on offerings available to them, and ultimately help them achieve better outcomes? This would help providers proactively engage with their patients over the long term, increasing the hospital’s brand loyalty while reducing costs—as opposed to reactively seeing patients as they need care.
- Clinical intake intelligence: How often have you sat at a doctor’s office with a clipboard and pen in hand, already five minutes late to your appointment but still needing to complete a basic questionnaire? There’s been an effort to digitize this experience. Health Note takes it to the next level by sending patients a digitally powered (i.e., via SMS) dynamic questionnaire: the next question changes based on their responses to the previous question. Patients answer the questions before their visit, mirroring what a doctor would ask in the first five minutes of the actual visit. The solution not only saves time for the front desk administrator but also for the doctor whose clinical note is already halfway autogenerated at the time of the visit.
- Streamlined workflow processes: Both generative and predictive AI can be important tools for doctors, nurses, and other skilled medical professionals who face a looming labor shortage and burnout. Machine learning algorithms can analyze vast patient data to predict hospital admissions and optimize staffing and resource allocation. Natural language processing streamlines documentation, while AI-powered scheduling systems minimize wait times and maximize facility utilization. Predictive maintenance for medical equipment prevents costly downtime.
- Coding automation: Empowered providers get paid faster and bill more accurately using AI models to autogenerate a billing code based on an unstructured doctor’s note. SignalFire led the Series A in CodaMetrix, which has a unique competitive advantage in this space, having spun out of Mass General Brigham—making it a data moat around high-quality training data (read more about our investment here).
- Clinical decision support: Natural language processing (NLP) and large language models (LLMs) can now extract key information from unstructured clinical notes and patient records. Having access to the entire patient medical record plus an AI tool for making sense of all this data enables more precise diagnoses and real-time intervention at a higher accuracy than what humans can accomplish alone. Where we see great potential is in tools that can assist clinicians, not replace them (e.g., providing a second set of eyes), speeding up diagnosis time by providing an assessment that a clinician can review to make a better-informed treatment plan. For example, SignalFire portfolio company Recora’s virtual cardiac platform is able to surface to providers who is more likely to have another heart attack after only a few virtual visits. The overall adoption of these models is relatively limited today and typically needs outpatient monitoring data, which can be provided by integrated continuous management systems like all.health, also in our portfolio.
- Machine learning and predictive analytics: Machine learning and predictive AI aren’t new to healthcare, but GenAI is renewing interest and unlocking new capabilities. Predictive analytics can identify the likelihood of future outcomes based on historical data. It requires analyzing complex medical data to detect anomalies, predicting patient deterioration, flagging the need for interventions for high-risk patients, personalizing treatment plans, and forecasting their efficacy. While most surveyed doctors see AI's potential, 78% want more information on how AI works in the medical context before allowing it to inform treatment plans.
- Medical robotics: The healthy 16.6% compound annual growth rate (CAGR) we see in the medical robotics market reflects the increasing use of AI-powered solutions in healthcare. Surgical robots, like Intuitive Surgical's three-decade-old da Vinci systems, provide minimally invasive procedures with greater precision for faster patient recovery times and reduced complications. AI algorithms enhance these robots' capabilities, allowing them to adapt to individual patient needs and learn from aggregate data across multiple cases. There are also new solutions like Diligent Robotic’s Moxi, an assistant that completes non–patient-facing tasks.
3. AI and Big Data for payors: Healthcare efficiency at scale
AI has the potential to enhance care while aligning with payors’ business models—effectively an insurance business—by reducing the cost of treatment while improving outcomes. We’re particularly interested in how AI can scale care from limited providers to huge populations, ensure patients stick to their treatment plans, and reduce overspending:
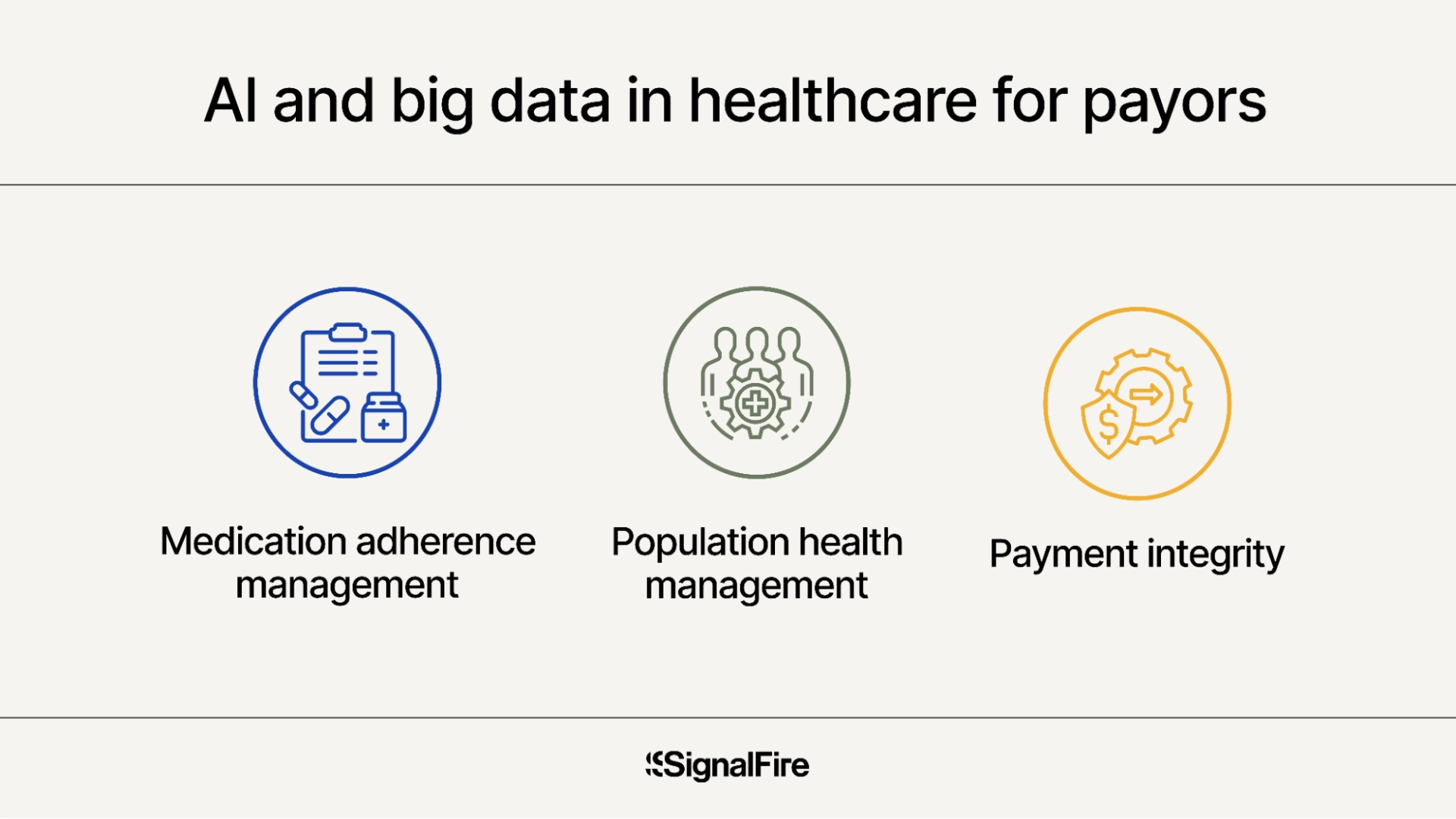
- Population health management: Every payor typically manages hundreds of thousands to millions of lives. Because they’re ultimately responsible for paying the bill, it’s important they understand how healthy their population is and which segments would benefit from proactive health management. Data-driven solutions include SignalFire portfolio company Color Health, which ropeviews the entire patient population’s data across all attributes and helps patients find the appropriate care they need.
- Medication adherence and management: The entire payor ecosystem pays an estimated $300 billion annually for medications that aren’t consumed, more expensive medications vs. generic equivalents, and medications that patients no longer need. Better data can help create a fuller picture of a patient’s existing conditions and engage with them in a highly personalized way, using behavioral economics principles to nudge them to take the right medicine at the right time. It’s why we invested in Wellth, a leader in leveraging behavioral economics to drive adherence to prescribed care and treatment plans.
- Payment integrity: Annually, claims waste, fraud, and abuse cost $200–300 billion. Ninety percent of the time, the reason payors overspend on claims comes down to human error—the person on the provider side has made a mistake and asked for more money than they should collect for a visit. The autonomous coding solution from CodaMetrix not only directly addresses this problem but, with increased adoption, could establish a common language that would allow payors and providers to transact equally and fairly.
4. AI and Big Data for pharma: Catalyzing new medicines
On average, Pharma spends over $1 billion and 10 years for a successful drug to come to market. Any data-driven and AI solutions that can expedite the drug development timeline or reduce costs are highly attractive to pharma, and they could enhance the length and quality of patients’ lives:

- Data for drug discovery: For researchers to identify what drugs to develop, pharma and life sciences companies need sufficient biological samples and clinical data. This can help them understand the most prevalent, dire, and costly maladies as well as how to fight them. Access to anonymized patient data can create a high-level view of conditions, and it can allow for subsequent deep dives into the patient journey for those living with a given condition and undergoing different treatments. A deep, valuable dataset like Ovation can help more quickly identify which drugs to develop. On the financial side, startups like SignalFire portfolio company Auxilius can optimize clinical trial management to make collecting sufficient data more efficient.
- Deep learning techniques: Drug discovery is a notoriously expensive process, with global R&D costs reaching over $260 billion USD in 2023. Deep learning techniques and generative AI can accelerating drug development by analyzing data sources and predicting molecular interactions. Major pharmaceutical companies are also exploring how recurrent neural networks can process longitudinal patient data to detect patterns indicative of adverse drug reactions much earlier than human observation. AstraZeneca seems to be ahead of the curve on this front, having added the first two AI-selected drug targets to its portfolio in 2021.
- Synthetic clinical trial arm: Real-world evidence data helps pharma recruit the most relevant patients for clinical trials and build a “synthetic control arm”—instead of collecting data from patients recruited for a trial who have been assigned to the control group, synthetic control arms model those comparators using data, saving pharma both time and money on the other half of these lengthy and expensive trials.
- Post-FDA targeting: After a new drug has passed stage-four approval—allowing it to be marketed—the question is, which is the best target market? Data can help identify key opinion leaders, as well as the prescribing physicians for patients whose profile is a match for the drug.
5. AI and Big Data for patients: Affordable, transparent care
Accessibility, affordability, and quality of care are constant concerns for all patients. While AI may make the whole industry more efficient, what matters most is that it could help heal more sick people faster and cheaper. Here are several ways that improved data insights and availability can help us all:
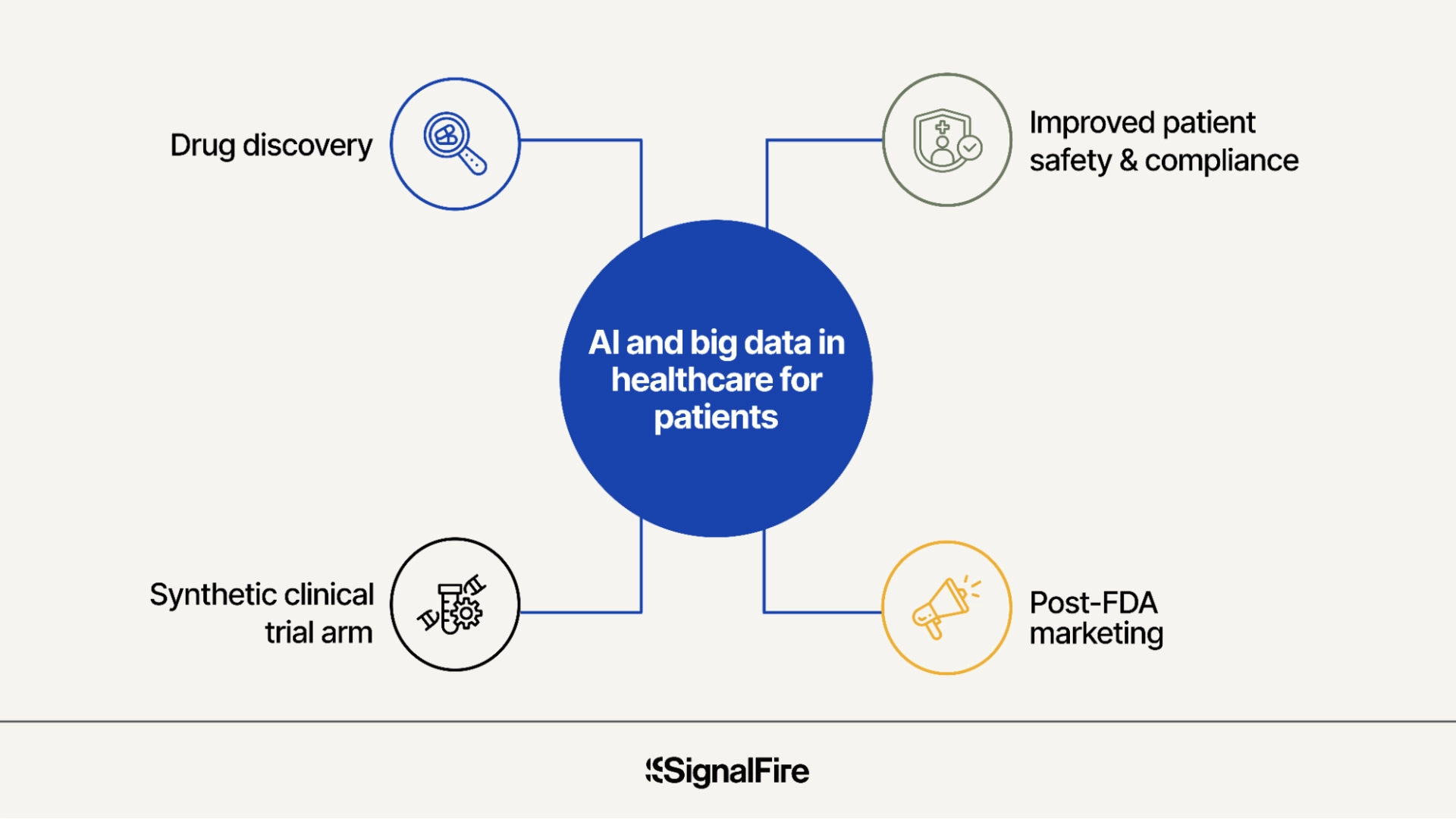
- Individual medical record access: Patients with chronic and rare diseases are currently tasked with manually assembling their information to get the best treatment possible. Currently under TEFCA, only certain use cases of data sharing are approved—a provider can pull information if they’re treating a patient, but a patient cannot directly pull information on themselves. We think an individual use case is going to be unlocked in the next year, helping everyone from the overburdened patient with clinical illness to the person who’s simply trying to keep track of their immunization records.
- Patient payments: Better data can help patients afford their healthcare. SignalFire portfolio company PayZen uses large amounts of patient data—spanning medical history, demographics, frequency of visits, and more—to provide patients with a personalized medical bill payment plan that has a 0% interest rate and zero fees.
- AI-powered image analysis: AI-powered image analysis is transforming patient care across multiple medical specialties. In radiology, deep learning algorithms can detect subtle abnormalities in X-rays, MRIs, and CT scans, often outperforming human radiologists in speed and accuracy. For example, AI tools can identify early-stage lung cancers or brain tumors that might be missed by the naked eye. In dermatology, machine learning models analyze skin lesion images to detect melanoma with high precision. Ophthalmology benefits from AI systems that can diagnose diabetic retinopathy from retinal scans. These AI applications not only improve diagnostic accuracy but also enable earlier interventions, leading to better patient outcomes and potentially life-saving early detections.
Challenges of AI in healthcare
“Growth at all costs” has no place in healthcare. The high stakes and tight regulations introduce added moral and technical complexity. Some critical obstacles for founders to proactively navigate include:
- Ethical concerns: Ensuring patient privacy and trust is paramount—especially with the rise in AI-powered fraud. Startups must implement robust data security measures and keep data safe from ransomware attacks. They should also consider partnering with established healthcare institutions to create transparent data usage policies.
- Privacy risks: With 65% of organizations citing data privacy as a top concern for generative AI, startups must prioritize compliance with regulations like the Health Insurance Portability and Accountability Act. This may require significant investment in secure infrastructure and regular audits, potentially slowing down the product development cycles.
- Bias: AI algorithms in healthcare have been found to exhibit racial and other biases, which can lead to disparities in patient care. Startups must proactively address this by diversifying their training data, implementing bias detection tools, and regularly auditing their AI models for fairness.
- Workflow disruption: Introducing AI tools often requires healthcare providers to change established processes. Startups should focus on creating intuitive, user-friendly interfaces and providing comprehensive training and support to ease the transition and encourage adoption.
- Data quality: In healthcare, poor data quality can have life-or-death consequences. Startups must invest in robust data cleaning and validation processes, potentially partnering with data quality assurance experts or developing proprietary data verification algorithms.
To overcome these challenges, founders can:
- Collaborate with healthcare institutions and regulatory bodies early in the development process
- Invest in building diverse, interdisciplinary teams including healthcare professionals, data scientists, and ethicists
- Develop clear, transparent policies on data usage and AI decision-making processes
- Implement continuous monitoring and improvement cycles for their AI models
- Focus on creating demonstrable value and ROI for healthcare providers to justify the disruption of implementing new technologies
By addressing these challenges head-on, startups can build trust with healthcare providers and patients, paving the way for wider adoption of AI-powered healthcare solutions.
Building for healthcare? We want to hear from you
If you’re working on a company in the health and pharmatech data, analytics, and AI space, please reach out to me, YY (Yuanling Yuan), and my fellow SignalFire partner Sooah Cho.

At SignalFire, we like to say, “Think of us as an extension of your team that scales with you.” Beyond our in-house Beacon AI for assistance with recruiting and sales, we offer a range of in-house experts and deeply connected industry advisors to help you solve problems and open doors.
By unlocking the secrets trapped within our medical data, we can build a healthier future for everyone.
*Portfolio company founders listed above have not received any compensation for this feedback and may or may not have invested in a SignalFire fund. These founders may or may not serve as Affiliate Advisors, Retained Advisors, or consultants to provide their expertise on a formal or ad hoc basis. They are not employed by SignalFire and do not provide investment advisory services to clients on behalf of SignalFire. Please refer to our disclosures page for additional disclosures.
Related posts

Why we’re continuing to invest in Tofu’s vision - Reducing martech bloat for GTM teams
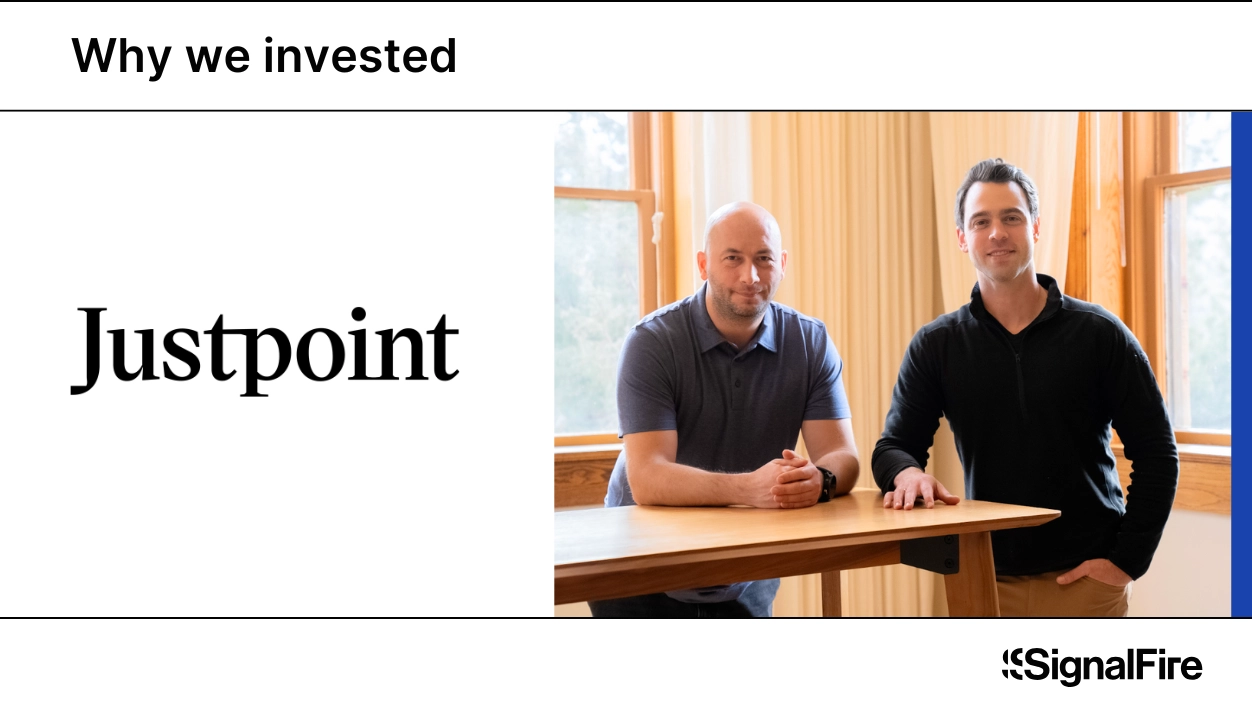
Justpoint raises $95M to tackle the toxic exposure epidemic with AI
