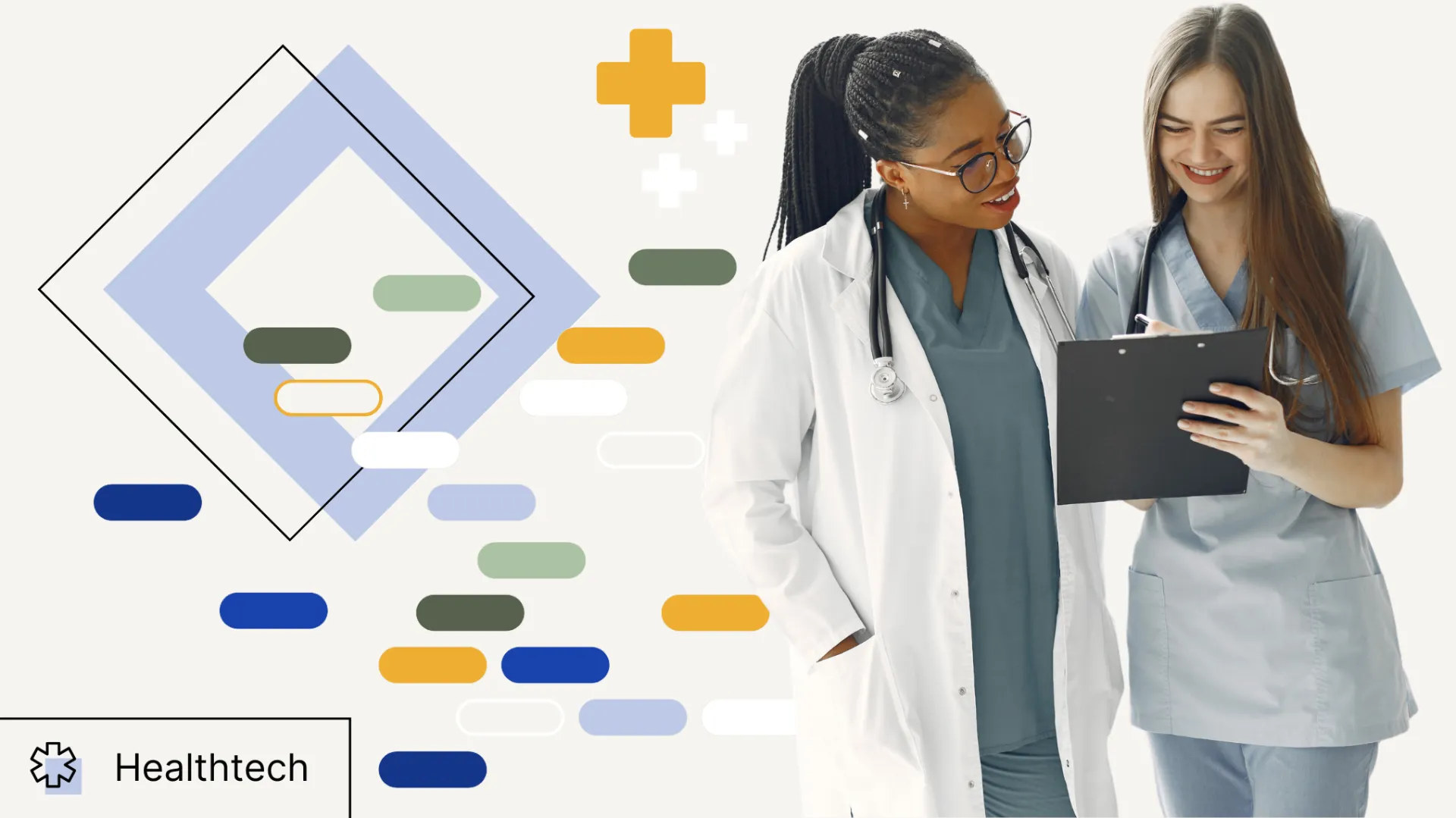
Nowhere will AI's impact be more obviously transformative than in healthcare. That's no exaggeration, given the long list we’ve shared in the past of healthcare industry problems AI can solve. Despite the best efforts of millions of brilliant minds, massive gaps remain in healthcare today. But AI is coming to help, supporting physicians and researchers to:
- Diagnose patients earlier and more accurately, saving countless lives
- Engage every individual in a more personalized way, keeping them invested in their personal healthcare journey
- Discover new treatments and novel cures for conditions that have eluded scientists for decades
- Address issues regarding health equity and access to quality care
The only way to make those visions a reality is to provide the most novel machine learning models with enough data…to learn. Unfortunately, that isn’t as easy in healthcare as simply text-scraping the web. Most healthcare data currently sits siloed in individual health systems, unable to be used by cutting-edge models. Even when it’s well curated, regulations such as HIPAA and GDPR often present challenges to sharing patient data easily.
The key takeaways:
- AI will transform every aspect of healthcare delivery, but first, standardized and diverse data is necessary.
- Hospitals and health systems have gravitated toward federated environments to share data, but these pose challenges to model developers, who prefer the flexibility to curate and harmonize the underlying data.
- New technologies such as better de-identification techniques, hybrid data-sharing models, and better data preprocessing pipelines offer the ability to bridge this gap and unlock the value of healthcare data in a compliant and scalable way.
Health systems see a growing desire to leverage data to help patients
In recent years, academic medical centers (AMCs) and health systems have generated vast amounts of rich, useful data during routine care—spanning medical records, genomic datasets, imaging, lab results, and tissue samples. These systems have become increasingly interested in leveraging this data to develop AI tools alongside industry partners (including biopharma and startups) to speed up diagnoses, find new cures, and reduce medical errors. However, as they explore the potential uses of their data, they face a significant challenge—how to balance patient privacy, scalability, data fidelity, and the expectations of their technical partners. Just a few of their hurdles are:
- Standardization: Machine learning models typically require curation and harmonization of data to ensure only high-quality, clean data makes its way into the training set. Over the past decade, standards development organizations like Fast Healthcare Interoperability Resources (FHIR) and the Observational Medical Outcomes Partnership (OMOP) have created frameworks to standardize healthcare data—data-in-transmission and data-at-rest, respectively. While FHIR and OMOP have significantly improved data-sharing capabilities, they lack flexibility as the industry moves from single-source datasets to multimodal longitudinal datasets.
- Expense: Some types of data require expensive conversion processes to be valuable. We have seen a rapid increase in demand for endoscopic video and radiological imaging data, but these datasets come with significant costs for storage, IO, and compute for large health-system datasets.
- Regulatory requirements: Handling sensitive health data, governed by strict regulations such as HIPAA, requires AMCs and hospitals to be diligent in protecting patient privacy. This creates friction when trying to collaborate with external partners on data-driven projects, and it requires that data research either be conducted under IRB or conducted on data that has been certified as de-identified.
The shift to federated environments
Because of the highly sensitive nature of healthcare data coupled with the need to collaborate with other organizations, many AMCs and large hospital systems are turning to federated learning environments. Federated learning allows institutions to build and train machine learning models on decentralized data. In this model, data remains in the control of the healthcare provider, and only the insights (or trained models) are shared with external researchers or companies.
The federated learning approach offers several benefits:
- Enhanced data security: By keeping sensitive patient data within individual institutions, federated learning significantly reduces the likelihood of unauthorized access and potential security breaches that may occur with centralized data systems. Federated learning environments thus prevent unauthorized exfiltration of data, providing peace of mind to patients and healthcare organizations that their data is not being stolen or misused.
- Improved model performance: AI should be trained on ethnically and geographically diverse patient data. Because of the limitations of each provider's data, this often requires model developers to have access to data from multiple providers. Federated learning facilitates training on a broader array of data sources, which strengthens the accuracy and reliability of predictive models and makes them easier to generalize. For instance, models developed using varied datasets have demonstrated enhanced diagnostic performance in minority populations for conditions such as kidney and heart disease.
- Streamlined research collaboration: Federated learning encourages cooperation among various healthcare organizations, enabling them to collaborate on research projects without sharing sensitive patient information. Such partnerships can expedite drug development and clinical trials while maintaining ethical practices.
- Regulatory compliance: By implementing federated learning, health systems can meet strict regulatory standards for patient data protection while making data available for important research and analysis.
- Real-time monitoring and intervention: Federated learning can be used in real time to manage population health, allowing for the early identification of disease outbreaks and prompt interventions based on combined insights from various healthcare providers
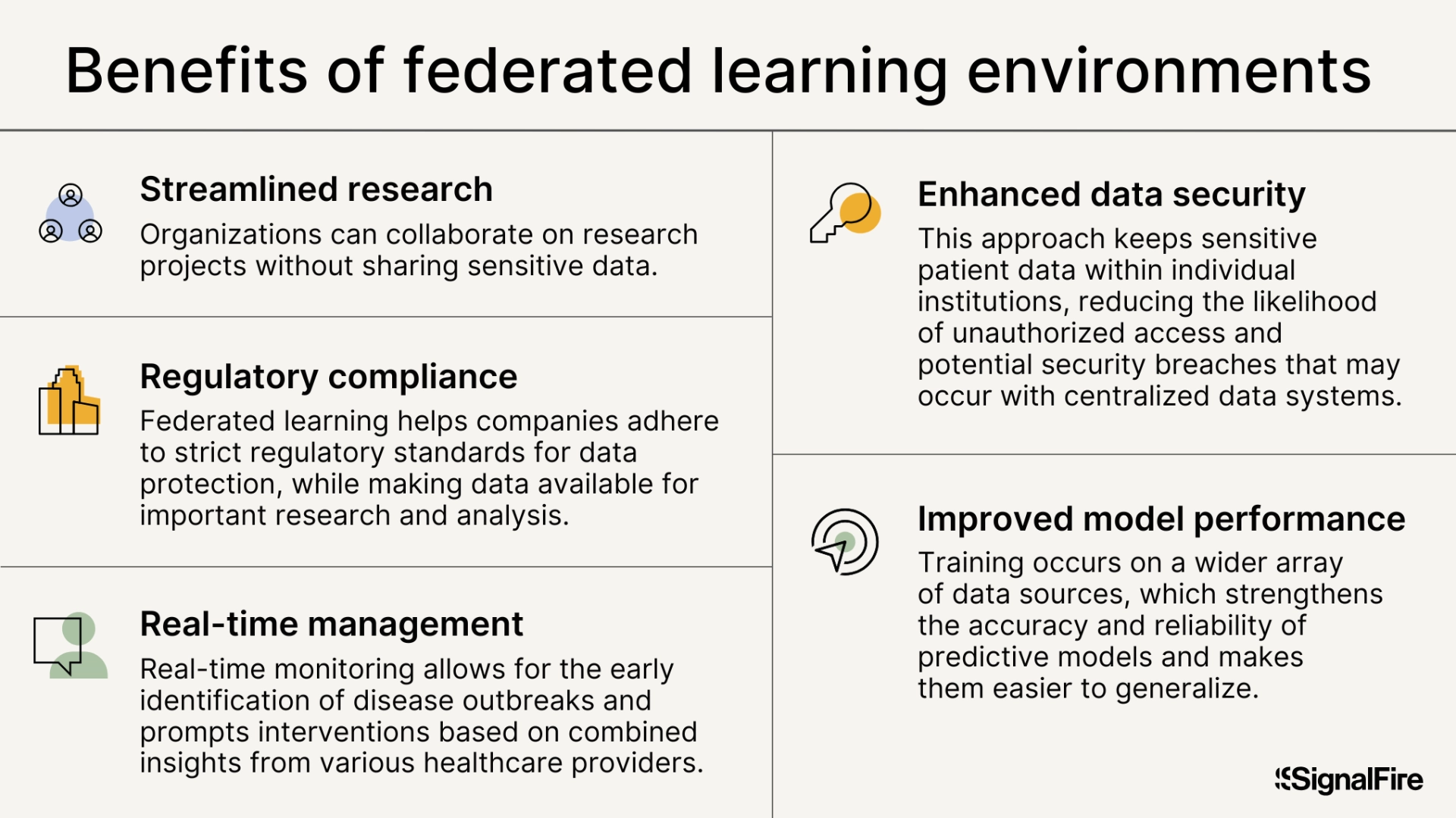
Even with all of these benefits, federated learning presents roadblocks for potential users of the data.
The lack of standardization increases the need for flexibility
Many organizations looking to collaborate with AMCs and hospital systems—particularly those interested in model development—have their own preferences when it comes to working with healthcare data. Technical teams often prefer full access to the underlying data to perform thorough data cleaning, harmonization, labeling, and transformation—steps critical to ensuring the data is in a format suitable for model development.
For instance, even after providers prepare medical data for use in a federated learning environment, the data is often messy and incomplete, containing errors, variations in terminology, and inconsistencies that can significantly hamper the performance of machine learning models. Thus, researchers and developers prefer to own the data preparation process in federated environments—but this is rarely allowed.
In a federated learning environment, customers generally do not have direct access to the underlying raw data, making it more difficult to perform the necessary cleaning and preparation. While many of the hosts of these federated environments aim to curate, label, and harmonize data in advance, the lack of true data standardization leads to inefficiency. Furthermore, without the ability to fine-tune their models on the raw data, the results may be less accurate or insightful, reducing the overall value of the data product. This creates tension between the data holders, who prioritize compliance and security, and the data consumers, who prioritize flexibility and access.
Finally, researchers’ and developers’ favorite tools may not be available for use in hosted environments. This includes TensorFlow, MATLAB, SAS, Stata, and R. Most federated environments have only a subset of the desired tools (typically just R). This may significantly limit developers’ ability to refine a model created outside a federated environment.
Building the holy grail
To bridge this gap, AMCs and hospital systems will need to work closely with their technical partners to find solutions that address both the compliance concerns of healthcare providers and the technical needs of data consumers.
Novel privacy-enhancing technologies (PETs) have shown promise, but none has proven to be the proverbial silver bullet. Trusted execution environments like secure enclaves (e.g., Intel’s SGX) offer hardware-level attestation for data security but continue to be vulnerable to side-channel attacks. Data sharing with secure multiparty compute can be extremely effective for simple tasks, but it doesn’t scale particularly well. Fully homomorphic encryption is orders of magnitude slower than other techniques—the list goes on.
However, there is hope that a combination of these techniques, along with some changes in process, can help cross the chasm. Some potential strategies include:
- Novel data de-identification technologies: Developing more flexible and automated approaches for expert determination de-identification, or generating data equivalents to de-risk data sharing using techniques such as differential privacy. Differential privacy uses machine learning to provide a verifiable mathematical guarantee that a resulting data set has been properly deidentified, thus making expert certification quick, easy, and less expensive.
- Hybrid models: Offering a hybrid approach that allows limited, controlled access to raw data in secure environments to third-party model developers for self-cleaning and harmonization while using federated learning for model training.
- Data preprocessing pipelines: Developing standardized preprocessing pipelines that de-identify, clean, and harmonize the data on the institution’s side, providing customers with ready-to-use datasets that meet their technical requirements. This can be accelerated via data standards like OMOP CDM, OpenEHR, and CDISC SDTM.
- Improved collaboration tools: Investing in collaborative platforms that allow technical teams to interact with data in ways that meet both compliance and research needs, such as sandbox environments where data can be viewed but not exported.
- Expanded toolsets: Making a broad range of toolsets available in FL environments to cater to a broader range of developers.
We at SignalFire believe the next generation of healthcare data sharing—critical to the success of healthcare AI efforts—will require a blended approach: leveraging federated learning where possible but sharing raw data when necessary using a combination of PETs and data security mechanisms to provide assurances against exfiltration.
It remains an open question how this will be accomplished. Do we see industry collaboration to create a “United Nations” for healthcare data? Or do a set of innovators create marketplaces for standardization, competing on speed and usability?
If you’re a founder building in the area of data collaboration in healthcare, we’d love to speak with you. SignalFire invests heavily in the healthcare technology space, from pre-seed to Series B. Reach out to healthcare@signalfire.com or contact Sahir or Tony!
*Portfolio company founders listed above have not received any compensation for this feedback and may or may not have invested in a SignalFire fund. These founders may or may not serve as Affiliate Advisors, Retained Advisors, or consultants to provide their expertise on a formal or ad hoc basis. They are not employed by SignalFire and do not provide investment advisory services to clients on behalf of SignalFire. Please refer to our disclosures page for additional disclosures.
Related posts
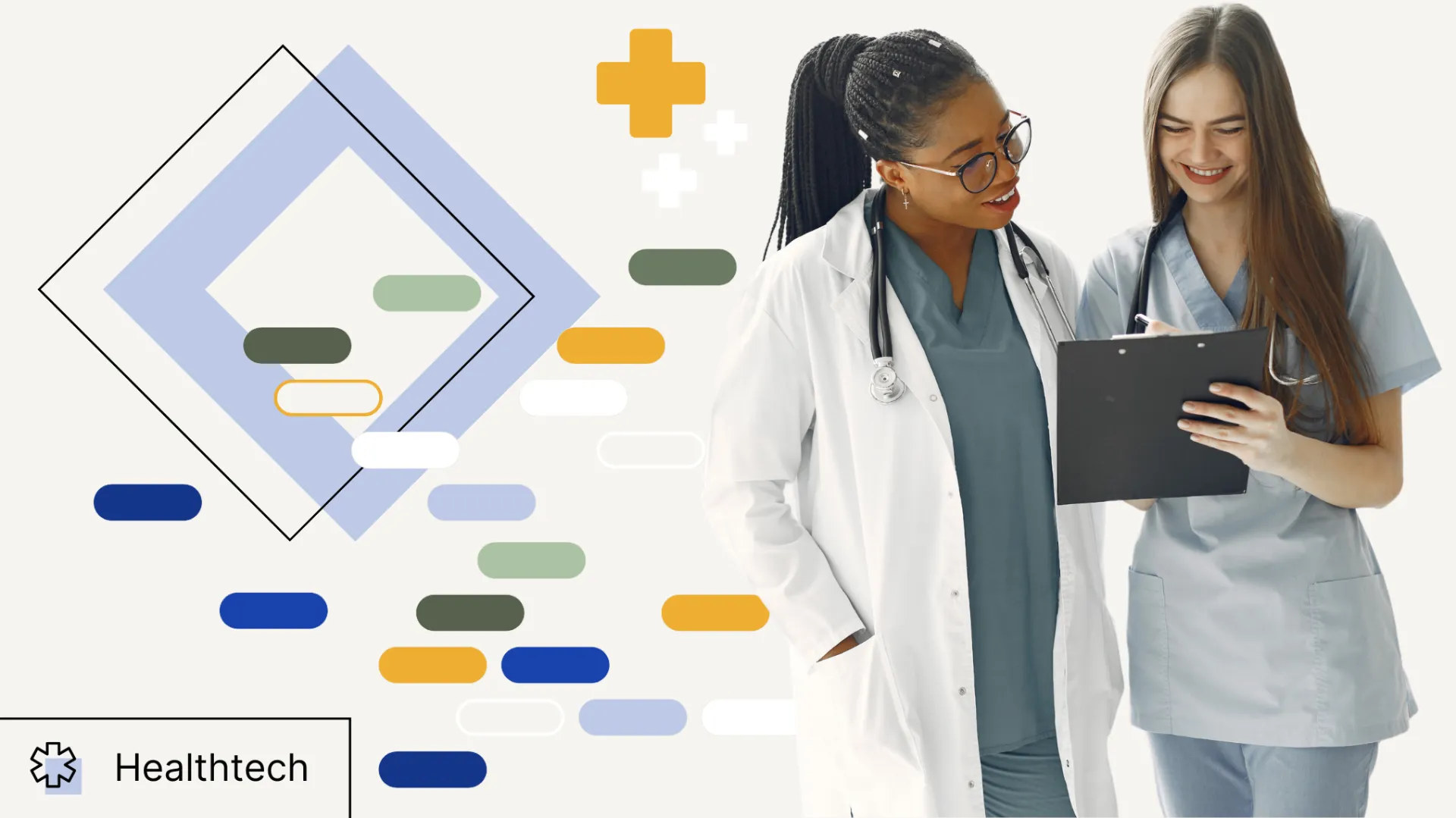